fig-chapter7
正在显示
This source diff could not be displayed because it is too large.
You can
view the blob
instead.
This source diff could not be displayed because it is too large.
You can
view the blob
instead.
Book/Chapter7/Chapter7.tex
0 → 100644
This source diff could not be displayed because it is too large.
You can
view the blob
instead.
Book/Chapter7/Figures/figure-MASS.tex
0 → 100644
Book/Chapter7/Figures/figure-bpe.tex
0 → 100644
Book/Chapter7/Figures/figure-data-clean.tex
0 → 100644
Book/Chapter7/Figures/figure-encoder-fin.tex
0 → 100644
Book/Chapter7/Figures/figure-main-module.tex
0 → 100644
Book/Chapter7/Figures/figure-norm.tex
0 → 100644
Book/Chapter7/Figures/figure-unk-of-bpe.tex
0 → 100644
Book/Chapter7/Figures/figure-wmt16.tex
0 → 100644
Book/Chapter7/Figures/figure-word-change.tex
0 → 100644
Book/Chapter7/Figures/figure-word-root.tex
0 → 100644
Book/Chapter7/Figures/learning-rate.tex
0 → 100644
Book/ChapterPreface/ChapterPreface.tex
0 → 100644
Book/Chapters/Biblio-Database.bib
deleted
100644 → 0
Book/Chapters/bibliography.tex
deleted
100644 → 0
Book/Chapters/chapter1.tex
deleted
100644 → 0
Book/Chapters/chapter2.tex
deleted
100644 → 0
Book/Chapters/chapter3.tex
deleted
100644 → 0
Book/Chapters/index.tex
deleted
100644 → 0
Book/Chapters/titlepage.tex
deleted
100644 → 0
Book/Figures/010813165162.pdf.pdf
deleted
100644 → 0
File deleted
Book/Figures/fig-NEU-1.jpg
0 → 100644

102 KB
Book/Figures/fig-NEU-10.jpg
0 → 100644
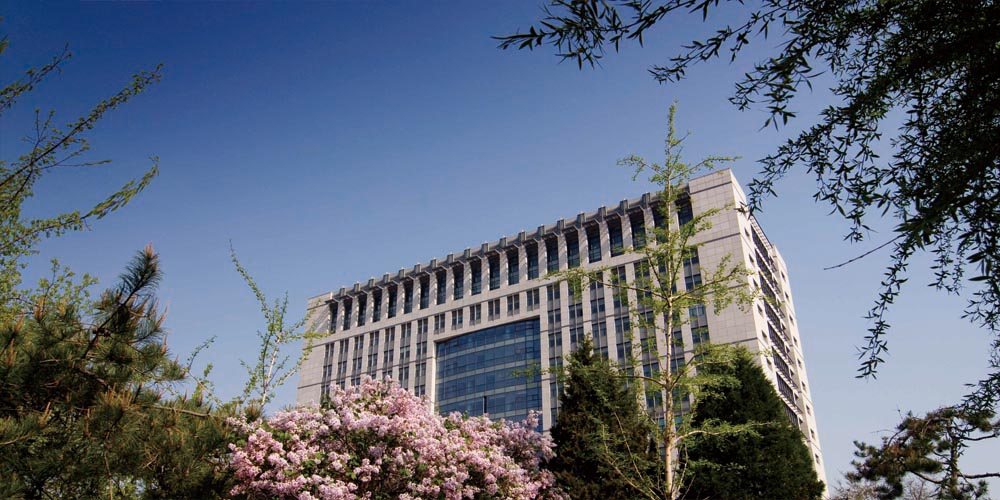
144 KB
Book/Figures/fig-NEU-2.jpg
0 → 100644

102 KB
Book/Figures/fig-NEU-3.jpg
0 → 100644

88.3 KB
Book/Figures/fig-NEU-4.jpg
0 → 100644

175 KB
Book/Figures/fig-NEU-5.jpg
0 → 100644

171 KB
Book/Figures/fig-NEU-6.jpg
0 → 100644

129 KB
Book/Figures/fig-NEU-7.jpg
0 → 100644

131 KB
Book/Figures/fig-NEU-8.jpg
0 → 100644

119 KB
Book/Figures/fig-NEU-9.jpg
0 → 100644

144 KB
Book/Figures/fig-cover.jpg
0 → 100644

222 KB
Book/Figures/placeholder.jpg
deleted
100644 → 0

19.8 KB
Book/mt-book-xelatex.ptc
0 → 100644
请
注册
或者
登录
后发表评论